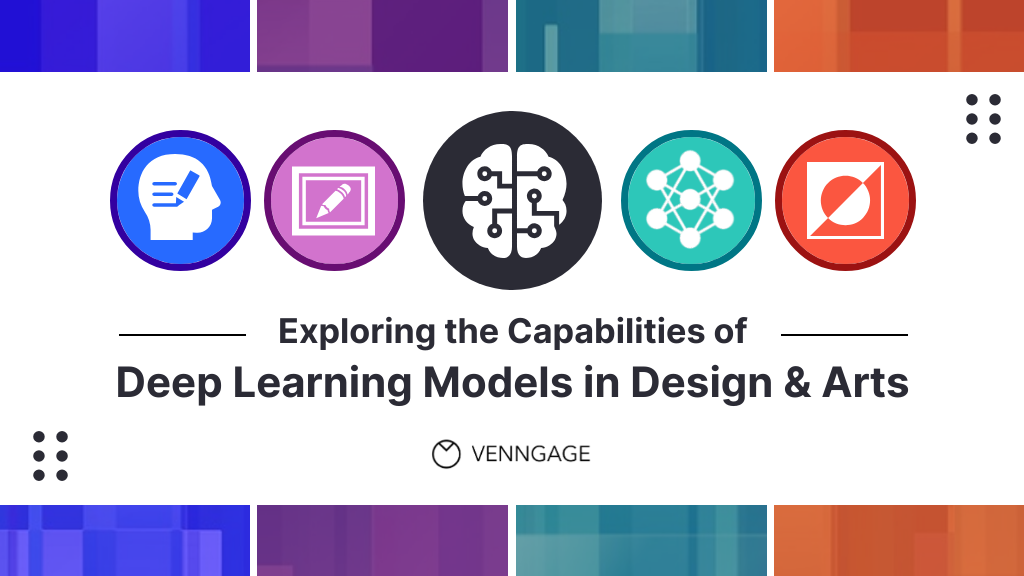
Deep learning models are revolutionizing the way we approach design and the arts, blurring the lines between technology and creativity.
But what are their capabilities and what do they actually solve?
In this article, I’ll talk about artificial intelligence and its subsets, making these advanced concepts easily understandable.
I’ll share insights on how AI is reshaping the tools and techniques in design and arts, highlighting its role in modern creativity.
By examining the unique features and problem-solving capabilities of various AI models, I aim to illuminate the types of groundbreaking designs they enable.
Join me to see how creativity is being redefined by the power of AI.
Understanding AI and its subsets
As we dive deeper into the intricacies of AI, it’s essential to understand its key subsets that are pivotal in advancing both design and the arts. These subsets, each with its unique capabilities,
are the cornerstone of how AI interprets, learns from and interacts with the world around us.
Let’s break them down:
NLP (Natural Language Processing)

Source: Freepik Stock Photo
- NLP refers to the branch of computer science—and more specifically, artificial intelligence (AI)—focused on enabling computers to understand text and spoken words in a similar way humans do. It is a subset of AI that includes aspects of computer science and linguistics, aiming to make human communication like speech and text comprehensible to computers.
CNN (Convolutional Neural Network)
- CNN, a deep learning neural network, is designed for processing structured arrays of data such as images. It’s a regularized type of feed-forward neural network that learns feature engineering autonomously via filter optimization. CNNs are particularly effective for image recognition and processing tasks, using multiple layers, including convolutional layers, pooling layers and fully connected layers.
RNNs (Recurrent Neural Networks)
- RNNs are a type of artificial neural network characterized by the directional flow of information between its layers. They are specially adapted for sequential or time series data. RNNs involve directed cycles in memory, enabling them to build upon previous network models with fixed-size input and output vectors
At the forefront: AI and deep learning models in AI design and arts tools & softwares

Source: Canva Stock Photo
Artificial Intelligence (AI) and deep learning models are increasingly shaping the tools and software used in design and the arts. Understanding the key technologies involved is crucial for grasping their impact and capabilities.
Each of these technologies offers unique capabilities in AI design and art, from language understanding and generation to image creation and style transfer. They are at the forefront of the evolving landscape of AI in creative fields.
Here’s a concise breakdown of each of them:
LLMs (Large Language Models)
- Definition: Large Language Models (LLMs) are a type of language model notable for their general-purpose language understanding and generation capabilities. They are a form of artificial neural networks, primarily transformers, trained on vast amounts of data to learn billions of parameters. This extensive training allows them to perform complex tasks like understanding, summarizing, generating and predicting content in human language.
- Technique: LLMs use deep learning techniques and operate on large datasets. They apply neural network techniques, specifically self-supervised learning, to process and understand human languages or text.
Diffusion Models
- Definition: Diffusion models are generative models in AI that learn to model data distribution from input. They are known for their capability in creating data similar to what they are trained on, utilizing the principles of Gaussian noise, variance, and differential equations.
- Technique: These models work by progressively adding Gaussian noise to destroy training data, then learning to recover the original data by reversing this noising process. They are particularly effective in generative tasks and can be applied to tasks like image search and content-based image retrieval. Diffusion models are often integrated with LLMs to create a more sophisticated design.
GANs (Generative Adversarial Networks)
- Definition: Generative Adversarial Networks (GANs) are a class of machine learning frameworks used in generative AI. They consist of two neural networks, a generator and a discriminator, which work in opposition to each other.
- Technique: In GANs, the generator network creates fake data that looks real, while the discriminator network tries to distinguish between synthetic data and real data from a training set. This adversarial process enables GANs to generate new data with similar characteristics to the training data.
NST (Neural Style Transfer)
- Definition: Neural Style Transfer (NST) refers to a class of software algorithms that manipulate digital images or videos to adopt the visual style of another image. This technology is widely used for creating artificial artwork from photographs.
- Technique: NST involves combining the style of one input image with the content of another input image to generate a new image. This is achieved through an optimization technique that blends two images—the content image and a style reference image—resulting in an output that looks like the content image but in the style of the reference image.
Design features & problems addressed by these advanced deep learning models
So now, let’s explore how technologies like Large Language Models (LLMs), Diffusion Models, Generative Adversarial Networks (GANs) and Neural Style Transfer (NST) are not only revolutionizing the way we approach design, but also addressing complex challenges within the field.
For each of these models, we’ll uncover their unique features, the specific problems they solve, and the types of images they are capable of generating.
Large Language Models (LLMs)
Large Language Models (LLMs) like GILL (Generating Images with Large Language Models) and DALL-E have made significant advances in image generation and multimodal capabilities, unlocking new large language models use cases for designers. Here’s an overview of their design features, problems they address and the types of images they generate:
Design features and capabilities of large language models.
- Fusion of text and image models: GILL fuses text-only LLMs with pre-trained image encoders and decoders, translating text embeddings into visual model spaces. This enables the generation of images, retrieval and multimodal dialogue based on text and image inputs.
- Efficient mapping for image generation: GILL proposes an efficient mapping network (GILLMapper) that grounds the LLM to a text-to-image generation model, enabling it to process longer and more complex language inputs effectively.
- Multimodal Input Processing: GILL can process arbitrarily interleaved image-text inputs, unlike typical text-to-image models. This includes outputting retrieved images, newly generated images and text in a coherent multimodal dialogue generation.
Problems addressed by LLMs in design
- Content generation: LLMs enable designers to effortlessly generate text for various applications like product descriptions and social media posts. This feature addresses the time-consuming nature of content creation.
- Language data analysis: Designers can use LLMs to analyze customer reviews and feedback, helping to inform design decisions and create more effective products.
- Generation of DEI language: LLMs assist in producing inclusive and diverse language, tackling the problem of biased language in design.
- User research and market analysis: These models analyze customer feedback and social media posts, providing valuable insights into user needs and market trends, aiding in the creation of user-centered designs.
- Assistance in the design process: LLMs can generate design concepts, color schemes, and typography choices, serving as a starting point for designers and streamlining their workflow. In some cases, by utilizing LLMs, many tools can create visual designs such as infographics in an instant without designing them from scratch.
- Mapping natural language to other domains: LLMs can interpret various forms of text input, from queries to contextual information, enabling their application in diverse domains such as smart home commands or UI design in tools like Figma. This feature addresses the challenge of translating user desires or moods into actionable commands or designs.
- Capability for creating more groundbreaking solutions beyond human-engineered designs: The extensive training data of Large Language Models (LLMs) enables them to discern patterns and connections that might elude human designers. This distinctive feature allows AI to propose materials or designs that enhance efficiency or outperform traditional human-created designs in terms of effectiveness. Utilizing AI’s prowess in revealing deeper insights from intricate data, engineers have the opportunity to investigate new design avenues
Types of images generated by LLMs
- Versatile styles and concepts: DALL-E can generate images in multiple styles, including photorealistic imagery, paintings and emojis. It can blend concepts creatively, often adding relevant details to images based on the context of the prompt.
- Visual reasoning and problem solving: DALL-E’s visual reasoning ability is sufficient to solve complex visual tests like Raven’s Matrices, often used to measure human intelligence.
Diffusion models
Diffusion models, particularly Stable Diffusion, represent a significant advancement in the field of AI-driven image generation. Below is an overview of their design features, the problems they address and the types of images they are capable of generating.
Design features and capabilities of stable diffusion
- AI-Powered image editing: Stable Diffusion is an AI technique used for creating high-quality images and performing image editing tasks like color correction and noise reduction. It involves training models using input images and refining the process until the network stabilizes.
- Text-to-image model: It is a deep learning model that generates detailed images based on text descriptions. It can also perform tasks like inpainting, outpainting and image-to-image translations guided by text prompts.
- Latent diffusion architecture: The model generates images by iteratively denoising random noise, guided by the CLIP text encoder. This process continues until a set number of steps are reached, resulting in an image that represents the trained concept.
Problems addressed by stable diffusion
- Iterative design development: Diffusion models enable making small iterative changes once a theme is liked. This is particularly useful for refining concepts without starting from scratch each time.
- Combination of different designs: Stable Diffusion allows the blending of elements from different designs. For example, the face of one car can be combined with the body of another, with the tool seamlessly integrating these elements.
- Open source and accessibility: Being open source and free, Stable Diffusion is accessible to anyone with a computer having 4GB of VRAM. This feature solves the problem of accessibility and cost associated with many design tools.
- Flexibility in design approach: The tool allows for a wide range of inputs, from sketches to 3D renderings, giving designers the flexibility to use various assets in their creative process.
- Enhanced creativity and idea generation: Stable Diffusion facilitates the generation of a multitude of design variations, speeding up the creative process and aiding in the exploration of diverse design possibilities.
- Generating product images: Stable Diffusion can create high-quality product images from text descriptions, offering an efficient alternative to traditional product photography.
- Interior design and architecture: It can restyle existing structures by simulating different textures, furniture styles or architectural facades. Converts basic SketchUp views into detailed renders, helping in visualizing architectural and interior design concepts and enhances rudimentary sketches into vivid, detailed visualizations, aiding in the design process from concept to completion.
- Web Design: Diffusion models can be integrated with ChatGPT to brainstorm content ideas and generate corresponding visuals and create detailed design briefs with ChatGPT and use them as prompts for visual generation.
Types of images generated by stable diffusion
- Detailed imagery from text descriptions: Stable Diffusion excels in generating detailed images from text descriptions. This includes creating new images from scratch or altering existing ones to incorporate new elements described in the text prompts.
- Diverse application: The model has been adapted for various use-cases beyond standard image generation, such as medical imaging and algorithmically generated music.
GANs (Generative Adversarial Networks)
Generative Adversarial Networks (GANs) are a prominent framework in machine learning, particularly known for their application in generative AI. Let’s look at its features, use cases, and the type of image it generates.
Core features of GANs
- Two neural networks; a generator and a discriminator: The generator produces new data, while the discriminator evaluates its authenticity. This indirect training approach, where the generator aims to fool the discriminator, allows the model to learn in an unsupervised manner.
Problems addressed by GANs
- Generating realistic images: GANs are used to create highly realistic images, which opens up new possibilities for artists and designers to produce lifelike visuals without traditional artistic methods.
- Creating new art forms: GANs facilitate the emergence of novel art forms, blending human creativity with machine intelligence. This allows for the exploration of new artistic territories and the creation of unique artworks.
- Style transfer: GANs are effective in style transfer, enabling the transformation of an image’s visual style while preserving its content. This is particularly useful in fashion design and other fields where style experimentation is crucial.
- Image inpainting: GANs are employed for image inpainting, where they fill in missing or damaged parts of an image, making them useful for digital art restoration and photo editing.
- Fashion and textile design: GANs generate unique clothing designs and intricate fabric patterns, allowing fashion designers to explore new design possibilities and create original garments and textiles.
- Architectural design: In architecture, GANs generate alternative architectural concepts and blueprints, helping architects explore diverse design options and unconventional architectural ideas.
- Interior design concepts: GANs aid in generating realistic visualizations of interior spaces, assisting interior designers in visualizing concepts and making informed design decisions
Types of images generated by GANs
- Produce images of human faces
- Generating lifelike photographs
- Creating images of cartoon characters
- Converting images to different styles
- Generating front views of faces
- Creating new poses for human figures
- Simulating aging in faces
- Merging different photos
- Filling in missing parts of photos
- Generating 3d models of objects
NST (Neural Style Transfer)
Neural Style Transfer (NST) represents a groundbreaking approach in the intersection of artificial intelligence and artistic creation. Here’s a complete insight into the unique features of NST, design problems it addresses and the variety of images it can generate, highlighting its innovative impact in the field of AI-assisted image transformation.
Design features and capabilities of neural style transfer (NST)
- Combining content and style: NST is an algorithm that merges the style of one image with the content of another, creating a new image that incorporates the style of the first and the content of the second. This involves understanding the content as objects, shapes and overall structure, and style as textures, colors and patterns.
- Algorithm process: The NST algorithm involves selecting content and style images, utilizing a pre-trained Convolutional Neural Network (like VGG-19, GoogLeNet or ResNet50), and defining content and style loss functions. The generated image is initially based on the content image and then optimized to match the style image through a total loss function, often using an Adam optimizer. Additional visual filters may be applied to enhance visual quality.
- Control and customization: NST allows for control over the extent to which style and content are represented in the final image, through a single loss formula that balances style reconstruction and content reconstruction.
Problems addressed by NST
- Art: Widely used to generate unique and impressive artworks by combining styles of famous artists with various content.
- Gaming and films: Useful in creating new visual environments and effects without designing them from scratch.
- Apparel design: NST can be employed in the fashion industry as a creative tool for designers. By combining elements from different images, NST enables the creation of unique clothing designs. For example, the style of a painting or photograph can be transferred onto a garment, creating an innovative and visually striking design.
- Artistic style recognition and creation: NST algorithms can recognize artistic styles from various artworks and apply these styles to new images. This allows for the creation of new art pieces that blend the content of one image with the style of another, leading to unique and imaginative artworks.
- Interactive style transfer in fashion: NST can be used interactively to design clothes. Users can select a clothing image as a content image and an art piece as a style image, then apply NST to create a unique design that combines the style of the artwork with the shape of the clothes.
- Inspiration for professional designers: NST offers a valuable tool for professional designers to quickly generate new ideas and concepts. By experimenting with different styles and patterns, designers can explore new creative directions and find fresh inspiration.
- Revitalizing classic art in contemporary design: NST allows for the fusion of classic art styles with modern design elements. This can result in the creation of products or artworks that have a contemporary twist while retaining a classic feel.
- Enhancing user experience in design software: NST can be integrated into design software, providing users with advanced tools to experiment with different styles and enhance the visual appeal of their creations.
Types of images generated by neural style transfer (NST)
- Artistic image transformation: NST is commonly used for creating artificial artwork from photographs. This involves transferring the appearance or visual style of famous paintings or artistic styles to user-supplied photographs. Artists and designers globally use NST to develop new artworks based on existing styles.
- Feature transform-based stylization: Recent advances in NST involve feature transform-based methods, like the whitening and coloring transform (WCT), for fast stylization. These methods are not limited to a single specific style and allow user-controllable blending of different styles, offering more flexibility and creativity in image generation
List of design tools that utilize deep learning models
AI design tools that utilize deep learning models are becoming increasingly popular in the design industry. These tools use deep learning algorithms to analyze and understand visual data, allowing designers to create more complex and sophisticated designs
One of the main advantages of AI design tools that utilize deep learning models is their ability to learn and adapt to new design trends.
In this section, let’s look at some of the most popular AI design tools that utilize deep learning models so that you will have a better understanding of these AI design tools.
- Midjourney: Midjourney is an advanced AI image generator that uses text prompts to create artwork in various styles. It operates within Discord channels and offers personal image creation, prompt customer support and community engagement.
- DALL-E 2: Developed by OpenAI, DALL-E 2 generates realistic images and artwork from textual descriptions. It is known for creating surreal, hyper-realistic images and offers a user-friendly experience with initial free use.
- Adobe Firefly: Adobe Firefly is a generative AI tool for converting text to images, transforming sketches to pictures and rendering 3D models.
- Stable Diffusion: This AI tool converts written words into images and is completely free to use. It allows users to edit pictures with AI and is user-friendly, offering high-quality image results.
- DreamStudio: Based on Stable Diffusion technology, DreamStudio creates and enhances images efficiently. It offers rapid results and artistic inspiration
- Venngage’s DesignAI Infographic Generator: This tool simplifies infographic creation, automatically generating designs without templates. It’s in beta and offers a variety of editable, realistic infographic options. The free version allows up to 5 designs at a time.
- Uizard: Used by over 400,000 people, Uizard facilitates the design of websites and apps with templates and real-time collaboration.
Related: Best AI Design & Art Generators You Need to Know [2024]
Final thoughts
It’s clear that the fusion of AI with creative disciplines is not just a trend, but a transformative movement reshaping how we conceive and execute artistic and design work.
These technologies are not merely tools; they are collaborators that bring a new dimension to creativity. They address complex design problems, generate diverse types of images and offer solutions that were previously unattainable. The remarkable features of these models reveal the depth of their potential in enhancing human creativity.
In embracing these technologies, artists and designers are not replacing their creativity but amplifying it, opening doors to uncharted territories of imagination and innovation. As we move forward, the synergy between AI and human creativity promises to unveil new horizons in the world of design and the arts.