In 2011, IBM showed off an AI system called Watson — a room-sized computer that wowed the world when it won Jeopardy, beating two of the game’s greatest champions.
Of course, this was more of a PR stunt than actual AI.
In a New York Times article, scientist David Ferrucci explained that, “Watson was engineered to identify word patterns and predict correct answers for the trivia game. It was not an all-purpose answer box ready to take on the commercial world. It might well fail a second-grade reading comprehension test.”
Simply put, Watson wasn’t a true, smart AI system. It was more like a trained parrot. It knew a lot of questions and answers, but it was only good for one task: answering trivia questions.
Fast forward a few years and researchers in academia and big tech companies, like Google, Facebook and Microsoft, began building large AI neural networks that could process billions of data points. These AI models weren’t trained to do specific tasks, like answer trivia questions. In other words, they were truly “smart.”
And they’ve grown smarter in leaps and bounds. In fact, the power of AI has grown over a thousand fold in the past two years alone. Now, with GPT-4 (OpenAI’s next generation model) dropping soon, AI is set to take its biggest leap yet.
Keep reading for a timeline infographic visualizing AI’s explosive growth, including GPT-4’s predecessors and competition.
Click to jump ahead:
- AI’s explosive growth [timeline infographic]
- A closer look at AI’s evolution
- Will GPT-4 achieve superhuman capabilities?
AI’s explosive growth [timeline infographic]
How do you quantify the power of an AI model?
One way is to look at the parameters the model is capable of. In an AI model, parameters are akin to variables — an AI learns these parameters through training and supervised learning. So, the larger the number of parameters, the more complex (or “smart”) it is.
This means we can see just how far this technology has come in the past few years by visualizing parameter sizes for AI models in a timeline template like this:
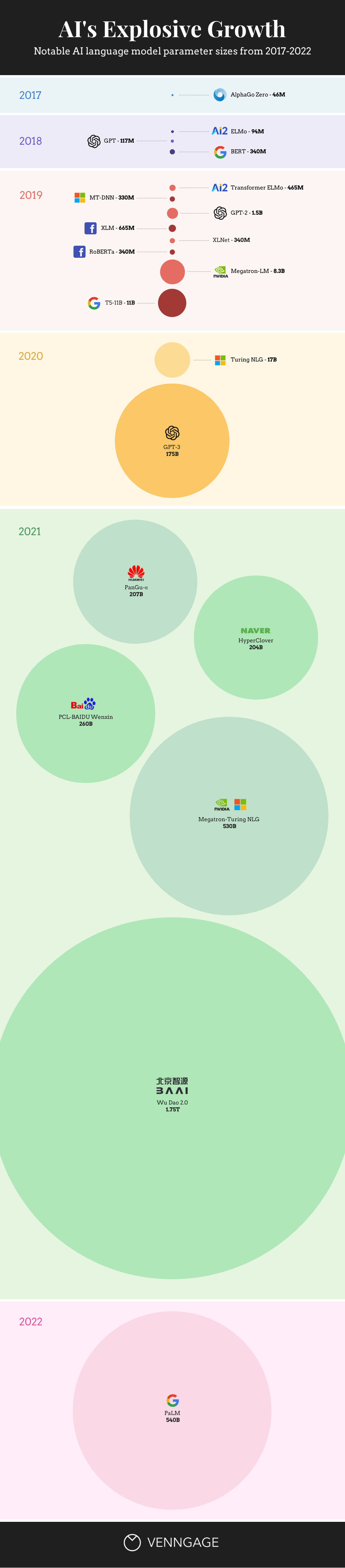
Note, we welcome sharing! You have permission to use this infographic on your blog or website. Simply copy the HTML code below to add this infographic to your site. Or, if you’d like to reference a specific statistic or fact, please include a link to this blog post as a source.
<img src="https://venngage-wordpress.s3.amazonaws.com/uploads/2022/10/AI-Growth-Timeline-Infographic-1.png" alt="AI's Explosive Growth [Timeline Infographic]"/><a href="https://venngage.com/blog/ai-growth">AI's Explosive Growth: Will GPT-4 Achieve Superhuman Capabilities [Timeline Infographic] </a> created by <a href="https://venngage.com/">Venngage Infographic Maker</a>
A closer look at AI’s evolution
Back in 2017, Deepmind (a Google company) released AlphaGo Zero: an AI model with 46 million parameters. This system was able to beat the world’s best chess champions after learning the game in only 4 hours.
Previously, AlphaGo (AlphaGo Zero’s predecessor) beat the world’s best “Go” player — an ancient Asian game more complex than chess. Ke Jie, the master Go player in question, called the AI “godlike.” Even back then, AI models were scary smart.
Then, in 2018, OpenAI released its first version of GPT. This model had 117 million parameters: more than double the number of AlphaGo Zero’s parameters.
OpenAI continued to break ground with the release of GPT-2 in 2019 — an AI model with 1.5 billion parameters. That’s 15x more parameters than its predecessor. But in the same year, NVIDIA released Megatron, an AI with 8.3 billion parameters.
Not to be outdone, Google and Microsoft released their own AI’s shortly after. Later in 2019, Google came out with T5-11B, an AI with 11 billion parameters. And Microsoft released Turing NLG in early 2020, an AI with 17 billion parameters.
But in June of 2020, OpenAI upped the ante with GPT-3, an AI with over 175 billion parameters. This outstripped GPT-2 by almost 200 billion parameters and brought with it one of the biggest breakthroughs in AI to date…
The AI scaling hypothesis
Some expected the vast increase in size of GPT-3 to lead to diminishing returns. On the contrary, the system continued to benefit as it scaled.
OpenAI’s researchers concluded:
“These results show that language modeling performance improves smoothly and predictably as we appropriately scale up model size, data, and compute. We expect that larger language models will perform better and be more sample efficient than current models.”
But GPT-3 didn’t just learn more facts than GPT-2, it was also able to infer how to do tasks from examples:
“It turned out that scaling to such an extent made GPT-3 capable of performing many NLP tasks (translation, questions answering, and more) without additional training, despite having not been trained to do those tasks — the model just needed to be presented with several examples of the task as input.”
You might say GPT-3 was the first truly smart AI. And the key was scale.

Google researchers explained this phenomena in reference to PaLM, an AI they released two years later: “As the scale of the model increases, the performance improves across tasks while also unlocking new capabilities.”
In sum, scaling AI models leads to smarter systems that are able to do more tasks. Thus, the AI scaling hypothesis:
“The strong scaling hypothesis is that, once we find a scalable architecture like self-attention or convolutions, which like the brain can be applied fairly uniformly … we can simply train ever larger NNs and ever more sophisticated behavior will emerge naturally as the easiest way to optimize for all the tasks & data. More powerful NNs are ‘just’ scaled-up weak NNs, in much the same way that human brains look much like scaled-up primate brains.”
…back to GPT-3 in 2020
GPT-3 is incredibly smart. It can follow examples and perform many tasks without being explicitly trained on them. For example, it can write articles as well as a journalist, translate text and come up with new ideas and funny names, or even tell a joke. An AI writer like GPT-3 can also be trained on specific topics or styles, allowing it to generate written content that is both high-quality and tailored to your brand’s voice and messaging. Furthermore, with advancements in AI content detection accuracy, the generated content can seamlessly align with your brand guidelines and ensure consistency across platforms.
The success of this large scale system paved the way for the massive AI’s that came next.
In 2021, Microsoft and NVIDIA joined forces to come up with Megatron-Turing NLG — an AI system with over 530 billion parameters, which they dubbed the biggest system to date. But not for long…
The Beijing Academy of Artificial Intelligence unveiled Wu Dao 2.0 in June of 2021. Another GPT-like language model, this AI boasts a shocking 1.75 trillion parameters. (Note, the limits of this AI have yet to be fully disclosed.)
And this year, Google unveiled their Pathway Language model (PaLM) with over 540 billion parameters, beating out Megatron-Turing NLG by a modest 10 billion.
Suffice to say, AI models have scaled up at an incredible rate in the last five years. The number of parameters these models boast has increased over 10,000 times.
Remember AlphaGo Zero with its 46 million parameters? It pales in comparison to Google’s latest AI and GPT-4 will likely be even bigger.
Will GPT-4 achieve superhuman capabilities?
How big will GPT-4 be? Some rumors suggest it will have over 100 trillion parameters, while others predict it will come in around five trillion. Either way, that’s magnitudes larger than the current biggest model.
If a genius described AlphaGo as godlike, just imagine what GPT-4 could be like.
My money’s on superhuman.